A Complete Guide on How to Build an ML Team
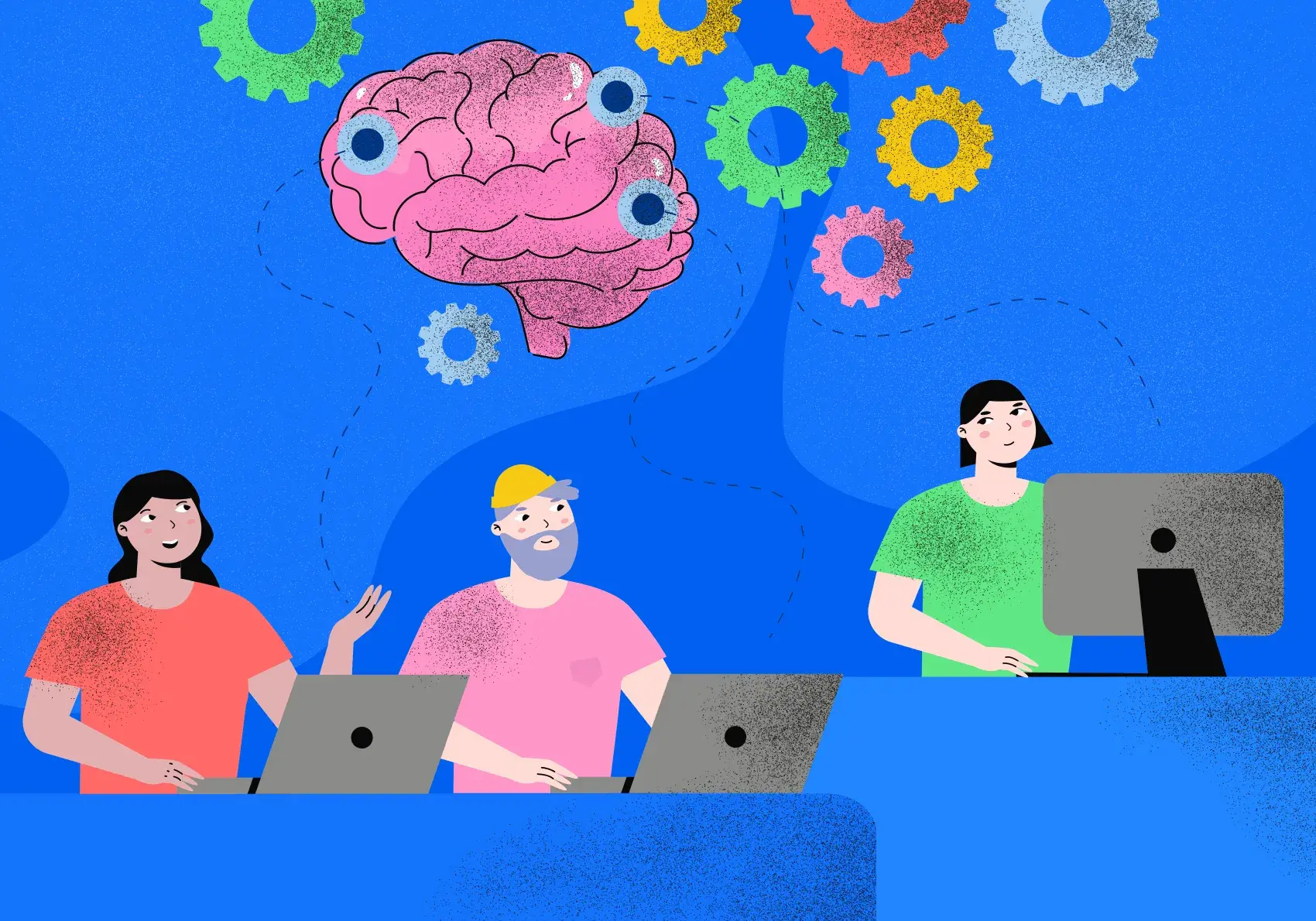
Machine learning technology is growing unprecedentedly, and many businesses are beginning to incorporate it into their products and services to become more competitive. However, building a machine learning team for AI projects can be challenging, especially as the field is relatively new and rapidly evolving.
Table of contents
- Why Build an ML Team for an AI Project?
- What Challenges You May Encounter When Building the ML Team
- How Roles Are Distributed Depending on the Stage of the ML Life Cycle
- Building the Right Machine Learning Team
- Hiring an ML Team: In-House vs. Outsourcing
- Examples of How ML Teams are Organized in Industry-Leading Companies
- Summing it all up
- FAQ
At Alternative-spaces, we recognize the crucial role that skilled machine learning teams play in developing innovative solutions for our clients. Our expert team has extensive experience building AI solutions and has helped many companies stay ahead of the curve by leveraging the latest machine learning technologies. In this article, we will share our expertise to guide you on how to build successful ML teams to drive AI projects and digital transformation.
We will cover essential aspects such as identifying the necessary skill sets, recruiting top talent, establishing an effective team structure, and fostering a collaborative and agile work environment that leads to successful ML projects.
Following our recommendations and guidance, you can build a Machine Learning team with the skills, expertise, and passion necessary to undertake complex AI projects delivering more significant business value and ROI.
Why Build an ML Team for an AI Project?
Machine learning is transforming industries and disrupting traditional business models. To stay ahead of the curve, companies that want to leverage the power of ML are building machine learning teams for their Artificial Intelligence projects. Here are the top 5 reasons why organizations should build an ML team for their AI projects:
1. Expertise – Machine learning is a specialized field that requires an in-depth understanding of algorithms, data science, and programming languages. Building an ML team means having access to the best minds in the area with the necessary skills and expertise to develop advanced AI solutions.
2. Innovation – Machine learning teams are tasked with developing new and innovative solutions that solve complex problems. Building an ML team creates a culture of innovation that fosters creativity and encourages out-of-the-box thinking.
3. Efficiency – Machine learning projects can be complex and time-consuming. Building an ML team ensures that projects are completed efficiently and within the required time frame, as the team works together to tackle challenges quickly.
4. Flexibility – Building an ML team allows companies to respond flexibly to market demands and changes. The team can adapt to new challenges and opportunities and develop AI solutions that meet evolving business needs.
5. Competitive Advantage – Machine learning is a competitive advantage. Building an ML team means gaining access to cutting-edge technology and expertise to help organizations stay ahead of the curve and remain competitive in the market.
What Challenges You May Encounter When Building the ML Team
Machine learning is a highly specialized field that requires a diverse set of skills and expertise. Building a successful ML team can be challenging, and different organizations face unique challenges.
Challenges for Startups
Startups often have limited resources, making it challenging to attract and retain top talent. Here are some additional challenges that startups may face:
- Lack of Experience – Founders of startups may need more experience building an ML team or developing AI solutions, which can make the process challenging.
- Competition for Talent – Startups compete with larger organizations for the same pool of talented individuals, often making it challenging to find the right fit.
- Cost Constraints – Hiring experienced machine learning professionals can be expensive, and startups may not have the financial resources to offer competitive compensation packages.
Challenges for Enterprises
Enterprises have more resources and existing processes but may face specific challenges when building an ML team.
- Cultural Resistance – Established enterprises may need more buy-in from existing employees who are resistant to change or new technological advancements.
- Siloed Departments – Enterprises have large and complex structures, often with siloed departments, which makes it challenging to streamline decision-making processes across teams.
- Data Management and Privacy Concerns – Enterprises collect vast amounts of data, which can create data privacy concerns. Building an ML team requires understanding data management and governance practices to ensure that sensitive data is used ethically and responsibly.
In conclusion, building an ML team requires careful consideration of various factors, including financial resources, experience, and talent acquisition. Whether you’re a startup or an enterprise, the key to success is developing a culture of innovation, collaboration, and flexibility that enables your team to produce cutting-edge AI solutions.
How Roles Are Distributed Depending on the Stage of the ML Life Cycle
Hiring a Machine Learning team means understanding the roles required for each stage of the ML life cycle. The roles and responsibilities of each team member vary depending on the project stage.
Here is an overview of how roles are distributed depending on the ML life cycle stage:
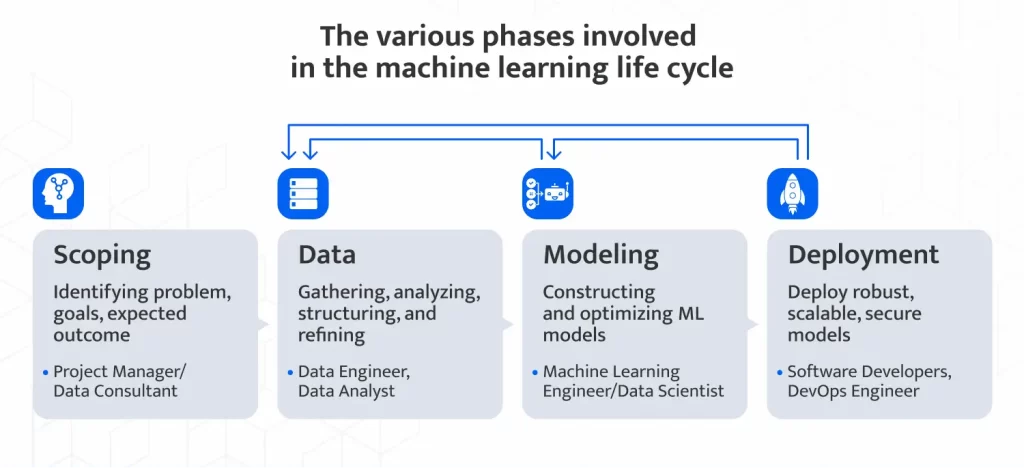
Stage 1: Scoping
The scoping stage involves identifying the problem to be solved, the project goals, and defining the expected outcome. These are the roles required for this stage:
- Project Manager or Data Consultant: Responsible for understanding the client’s business problem, formulating a data-driven solution, and managing the overall project.
Stage 2: Data
Data is the foundation of any ML solution. The data stage involves collecting, processing, and organizing data, and preparing it for analysis and modeling. These are the roles required for this stage:
- Data Engineer: Responsible for data collection, data preprocessing, and pipeline creation.
- Data Analyst: In charge of analyzing and interpreting data sets, identifying trends, and providing insights to the team.
Stage 3: Modeling
In the modeling stage, the team uses the prepared data to build and tune Machine Learning models. These are the roles required for this stage:
- Machine Learning Engineer or Data Scientist: In charge of selecting the best model for the problem statement, preparing data for modeling, creating and fine-tuning the model, and validating the model’s performance.
Stage 4: Deployment
Once the model is ready, it must be deployed in real-world operations. The deployment stage ensures that the models are robust, scalable, and secure. These are the roles required for this stage:
- Software Developers: Responsible for developing the application structure, infrastructure for model deployment, and testing.
- DevOps Engineer: Ensures the application deployment process is automated, scalable, and secure.
In conclusion, an effective ML team should have individuals with different backgrounds and expertise working collaboratively across different stages of the ML life cycle. The roles and responsibilities of each team member vary depending on the project’s complexity and the skills required for each stage. Understanding these roles is essential to building an efficient ML team that can deliver high-quality solutions.
Building the Right Machine Learning Team
Gathering a successful Machine Learning (ML) team is crucial to the success of any ML project. The following is a process for creating an effective ML team.
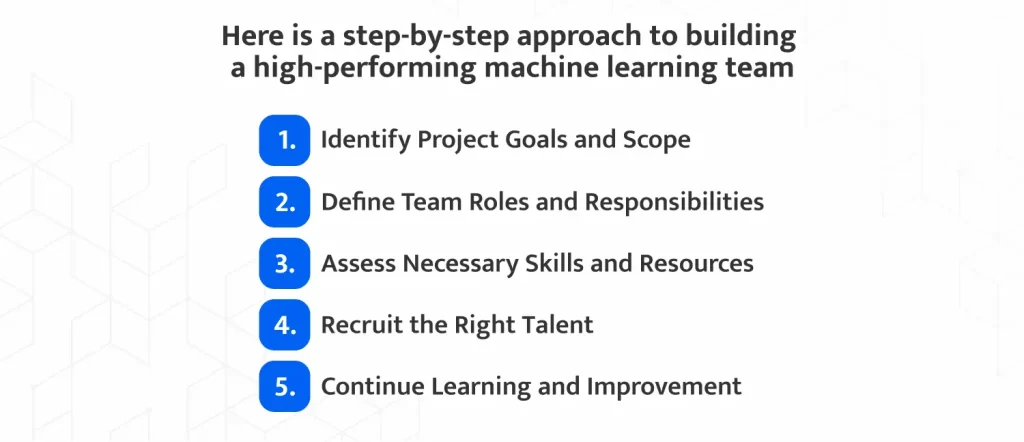
Step #1: Identify Project Goals and Scope
The first step in building an ML team is identifying project goals and scope. A clear understanding of business needs, problems, and expected outcomes is essential. It’s crucial to have buy-in from stakeholders and a clear indication of project feasibility before initiating any ML project.
Step #2: Define Team Roles and Responsibilities
The second step is defining team roles and responsibilities. Each member of the ML team, from project managers to data scientists, has different roles and responsibilities. Establishing clear communication channels and responsibilities for each team member ensures that everyone understands their role and that the project runs seamlessly.
Step #3: Assess Necessary Skills and Resources
The third step is assessing the necessary skills and resources. Understanding the skills and resources required to achieve the project goals is vital. The team should evaluate the organization’s current state, identify gaps, and assess the necessary skills and resources to fill the gaps. Standard ML skill sets include data analysis, statistics and probability, programming, machine learning techniques, and database experience.
Step #4: Recruit the Right Talent
The fourth step is recruiting the right talent. Organizations can recruit internal talent, contract to hire talent, or partner with a third-party provider specializing in ML. When recruiting, it’s essential to identify the specific skills required and thoroughly assess candidates’ portfolios, experience, industry expertise, and ability to work collaboratively.
Step #5: Continue Learning and Improvement
The last step is continuous learning and improvement. ML is a rapidly evolving field, and the team needs to develop and maintain expertise, experiment with new technologies, and improve communication and collaboration continuously. Providing ongoing training and development opportunities keeps the team’s skills current and relevant.
Building a machine learning team requires careful planning, organizational buy-in, and recruiting the right talent. By identifying project goals and scope and emphasizing continuous learning and improvement, organizations can build an efficient, effective, and successful ML team.
Hiring an ML Team: In-House vs. Outsourcing
When building a Machine Learning team, companies can either build an in-house team or outsource the work to a third-party provider. Here are some of the advantages and disadvantages of each model:
In-House Team
Advantages:
- Complete Control: Companies have full control over the development process, can execute on their timeline, and steer the project direction however they want.
- Cultural fit: In-house teams share the same culture, goals, and values of the organization, making project alignment more natural.
- Continuity: An in-house team allows the company to keep its in-house expertise and control development even after completing the project.
Disadvantages:
- Cost: Composing and maintaining an in-house team can be expensive, especially regarding talent acquisition, infrastructure, and additional project costs such as benefits and office space.
- Lack of diversity: For startup companies, hiring a team could result in a lack of diversity and experience in the team.
- Takes time: Composing an effective in-house team takes significant time and effort, delaying the project’s development.
Outsourcing
Advantages:
- Expertise: Outsourcing provides access to specialized knowledge and a team of experienced professionals in the latest ML trends.
- Flexibility: It provides flexibility in scaling the workforce according to business needs.
- Reduced costs: Outsourcing can lead to cost savings since companies don’t have to hire full-time employees, pay salaries, or provide office space.
- Faster time-to-market: Outsourcing helps expedite the ML development process, saving time and resources.
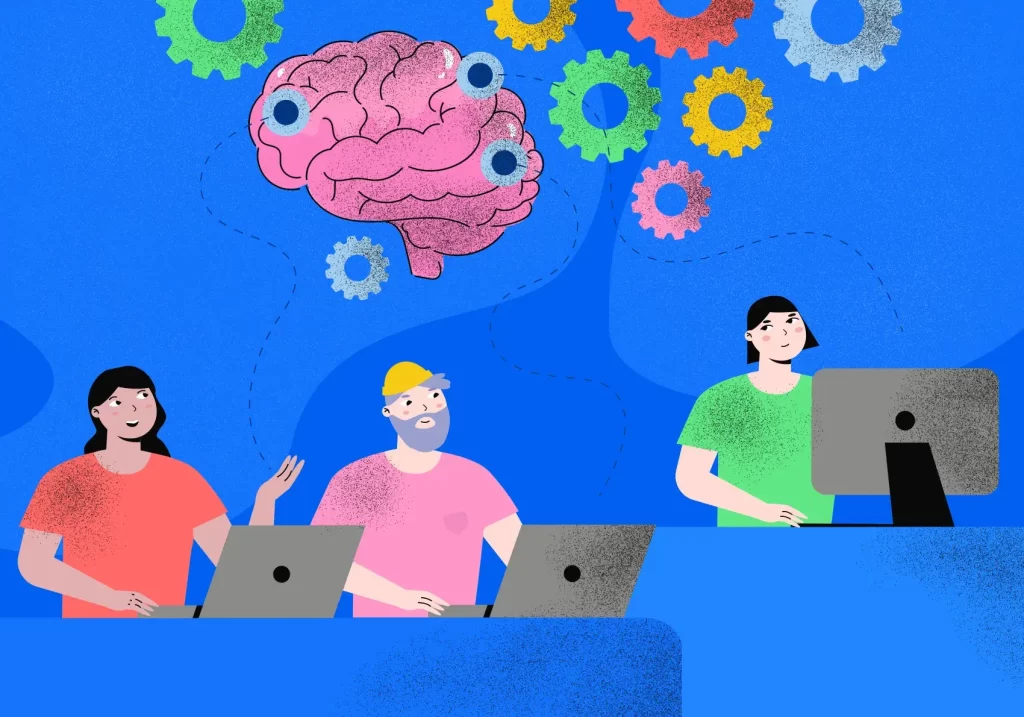
Disadvantages:
- Limited control: Companies may need more control over the development process than an in-house team, which may lead to difficulties in project alignment.
- Communication difficulties: Communication may be challenging when working with an outsourced team located in different parts of the world.
- Less cultural fit: The outsourced team may have different cultures, goals, and values than the company, leading to difficulties aligning project directions.
Outsourcing ML development can be an attractive option for many organizations, especially those that need more in-house resources or expertise to build an ML team from scratch. It can result in faster development, reduced costs, and access to specialized expertise. However, it’s essential to consider the disadvantages, such as communication difficulties and limited control over the project. By doing thorough research and identifying the goals, and overall project needs, you can determine whether an in-house or outsourced team will be the best option for their project.
Examples of How ML Teams are Organized in Industry-Leading Companies
Here are some examples of how popular companies have organized their Machine Learning (ML) teams:
Google’s ML teams are organized under Google Brain and Google Research, focusing on researching AI applications in already available products. The teams work on a large array of projects, apart from Google Search, Under Google AI, the company has divided its teams into several areas, such as Perception, Language, and Vision.
Facebook’s Applied Machine Learning (AML) assists in innovating products and experiences, designing and implementing breakthrough Machine Learning ideas, and driving-related research. The team works across all aspects of Facebook, including posts, photos, videos, and more. The team focuses on the development and deployment of state-of-the-art ML models.
Uber
Uber’s ML organizational structure is supported by UAI (Uber AI) Research and Development, which focuses on developing new algorithms, technologies, and architectures in the field of ML. UAI has teams working on fundamental, applied, and emerging research in AI and ML.
Amazon
Amazon’s Machine Learning teams operate under AWS AI and consist of hundreds of experts in ML, robotics, natural language processing (NLP), and computer vision. The teams at Amazon are divided as per different business segments and work closely with individual teams to design, develop, and deploy their machine learning models in their respective products and services.
Netflix
Netflix’s ML teams operate under the Netflix Research Team, which focuses on building and evolving a system that handles personalization, recommendations, and search at scale. Netflix’s ML teams concentrate on changing how the world experiences entertainment by revolutionizing and leading in recommendation systems.
In conclusion, various companies have organized their ML teams differently, depending on the unique requirements of their business and customer base. The teams’ size, structure, and focus can also vary depending on the company’s maturity and investment in machine learning.
Summing it all up
In conclusion, building a Machine Learning (ML) team requires careful consideration of project goals, identifying roles and responsibilities, assessing necessary skills, and recruiting the right talent. While companies can choose to build an in-house team or outsource to a third-party provider, they must decide which option suits them best.
Alternative-spaces has emerged as a leading IT provider that offers highly skilled and experienced ML professionals. Alternative-spaces’s AI and ML Teams are experienced in developing AI-based models and delivering AI and ML services tailored to specific client requirements. Contact our expert to discuss your ML project idea and we’ll definitely find the best solution and specialists for you.
FAQ
- Q: What machine learning roles should I consider for my team?
- A: There are several key roles you may want to consider when building an ML team. These include data scientists, machine learning engineers, data engineers, and product managers with expertise in ML.
- Q: What skills should I look for in potential team members?
- A: The specific skills you’ll want to look for will vary depending on the roles you’re hiring for, but some key skills include a strong foundation in programming languages like Python and a solid understanding of machine learning algorithms and techniques.
- Q: How can I assess a candidate’s technical skills during the hiring process?
- A: One great strategy is to ask candidates to complete a coding challenge or take-home project that demonstrates their skills. You can also use technical interviews to ask candidates targeted questions about specific concepts or scenarios related to machine learning.
- Q: How can I foster collaboration and communication within my ML team?
- A: Good communication is essential for any team, and it’s particularly important when building an ML team. To foster collaboration and communication, consider setting up regular team meetings and encourage team members to share their work and insights with each other. You can also consider investing in tools that streamline collaboration, like shared code repositories or project management software.
Content created by our partner, Onix-systems.
Source: https://onix-systems.com/blog/how-to-build-machine-learning-teams-for-ai-projects