How Artificial Intelligence (AI) Will Transform Your Business
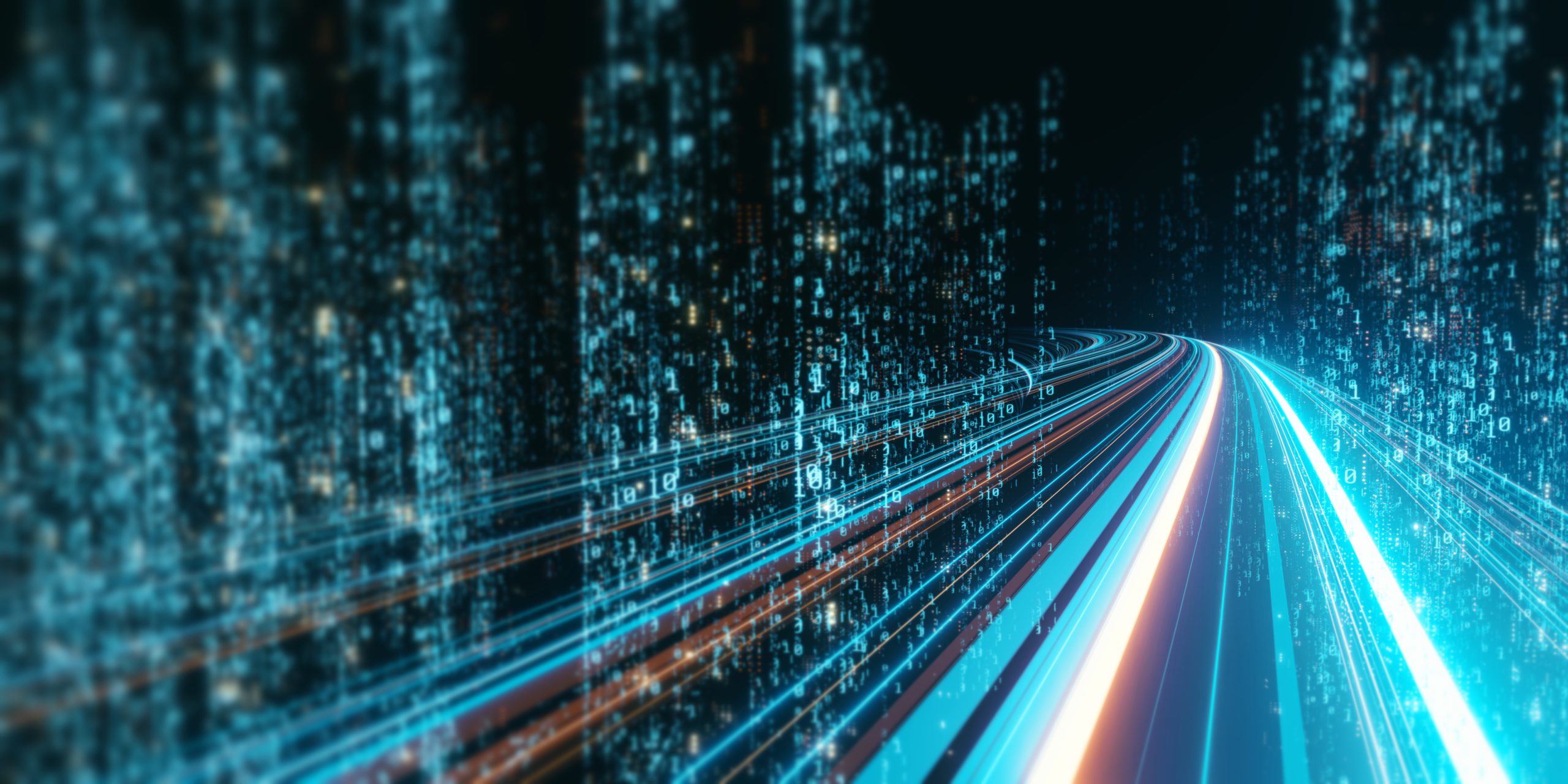
This article is for business owners and entrepreneurs wondering how artificial intelligence will transform business processes, whether they should invest in the technology, and what challenges they may face.
Alternative-spaces can advise you on integrating artificial intelligence (AI) in business operations and build a solution for your specific needs using our broad experience.
Table of contents
- Primary Areas of Application of Artificial Intelligence in Business
- The Challenges to Deploying AI for Business Needs
- Businesses that Have Transformed Their Operations with AI
- Conclusion: What Business Can Expect from AI in the Future
- Frequently Asked Questions
AI is basically a software form that can process and analyze massive amounts of data much faster and better than humans and even make decisions on its own. Although still associated with sci-fi thrillers, it is already a part of our everyday lives and virtually any industry.
According to IBM’s 2022 Global AI Adoption Index, the global AI adoption rate is 35%, a four-point increase from 2021. 42% of respondents are exploring the benefits of AI.
The integration of artificial intelligence in businesses occurs through off-the-shelf solutions powered by AI technology and embedding AI capabilities in existing operations across enterprises. 44% of organizations are working to embed AI into current applications and processes. Let’s review some of the most popular applications.
Primary Areas of Application of Artificial Intelligence in Business
Business intelligence
The ever-growing competition and customer expectations and complexity urge businesses to innovate continuously. AI can support their strategic planning and decision-making by generating actionable insights from customer data.
Businesses have long been trying to foresee consumer behaviors and market changes. AI can help to provide the answers. It can process billions of data points in real time or use historical data to project future outcomes with a high level of accuracy, enabling better-informed decisions.
These insights help companies increase their marketing effectiveness, create more personalized customer experiences, and manage their enterprises more effectively.
Banks, financial services, and fintech companies employ AI for predictive analytics and more niche applications, such as wealth management, trading decisions, fraud detection, and loan approvals.
Only some companies are ready to develop their own business intelligence systems and algorithms, so artificial intelligence-related business is sure to be in demand. For example, Microsoft’s Power BI provides its customers with software services, applications, and connectors that turn data from unrelated databases, webpages, or structured files into visually immersive and interactive insights. The integration of machine learning (ML) capabilities within Power BI can help businesses create ML models that make actionable forecasts and predictions and help automate business processes.
Customer relationships and customer service
The prevalence of online research, contactless options, hyper-personalization, comparison shopping, and other consumer trends drive more companies to adopt AI to streamline the purchasing journey and customer service.
Around 40% of larger companies, including companies in the automotive, chemicals, oil and gas, utility, and environmental sectors, use AI to create more personalized experiences, improve customer service agent productivity, and streamline the information search and FAQ for customers and employees.
Chatbots are one of the best-known AI applications in business. Even simple rule-based bots that provide pre-determined answers to specific questions faster than humans save time and resources. Intelligent bots use machine learning algorithms and natural language processing (NLP) to obtain information from customers, understand words and phrases, respond appropriately, and improve over time as they handle more conversations.
AI-powered chatbots combine the previous two types. They can conduct “sentiment analysis” of customer conversations and remember the context and the users’ preferences.
Consumers already actively communicate with Amazon’s Alexa, Apple’s Siri, Google Assistant, and other voice user interfaces. It’s easy to imagine that AI-powered digital assistants will soon be recognizing customers by face and voice across channels and partners. Customers will be able to resolve complaints in real time, place orders, and do anything that previously required interactions with human customer-care representatives. This will exponentially improve customer care accessibility and experience while reducing costs.
The financial sector is one of the best areas for this application of AI.
AI also helps companies generate automated business reports and conduct sentiment analysis of social media comments to understand people’s perceptions of their brand, products, and services.
AI systems can also assist human employees in serving customers, drawing on analytics and using “recommendation engines” of sorts to suggest next-best actions, how to take a conversation with the customer further, or how to present a specific offer. AI can also route customer calls not to randomly available representatives but to those best suited to handle a particular customer’s needs.
Customer relationship management (CRM) systems like Salesforce and Zoho require human intervention to remain up-to-date and accurate. AI can make these platforms self-updating, auto-correcting systems.
Personalized and targeted marketing
Some day, AI may become the ultimate search engine, understanding everything on the web, knowing what exactly users want, and giving it to them instantly. Meanwhile, machines already place advertisements on the web, designing them to optimize click-through rates.
Online search providers, online stores, and others use intelligent systems to understand consumers and their buying patterns. AI can use data from their online activities to predict their needs and interests, select optimal ads for specific products, and market particular products to particular consumers, increasing marketing efficiency and sales while reducing costs.
For retailers, AI technology enables personalized product recommendations based on consumers’ shopping and browsing history and predictive analytics.
Amazon, a pioneer in adopting deep learning for recommendation engines, has AI behind its homepage uniquely tailored to each of its 300 million users. Analyzing their browsing habits across the Internet, Amazon can better predict what they are most likely to buy and places selected products in a prominent position. By offering “frequently bought together” and “customers who viewed this item also viewed …” items, Amazon increases sales.
After an online shopper spends some time browsing products, clicking links, comparing options, and adding/removing items from the cart, the AI can generate valuable insights and re-target ads accordingly.
Social media and content streaming services also use AI to determine what content to offer to individual users first. This helps retain their interest and better satisfy their desires. For instance, Netflix is known to make AI-powered viewing recommendations and dynamically populate content that appeals to each user. Spotify monitors the users’ listening behavior and song preferences to suggest songs that each user will likely enjoy.
More efficient and cost-effective supply chains
Improved decision-making is a high priority for supply chain managers in all markets and industries. ML algorithms help forecast what will be needed, when, and the optimal time to move supplies. This helps minimize or eliminate overstocking and prevent a deficit of in-demand products.
Over 75% of commercial supply chain management applications are expected to deliver embedded AI, advanced analytics, and data science by 2026.
Retailers employ AI capabilities for intelligent store design, optimized product selection, monitoring of in-store activities and inventory on shelves, and controlling the freshness of perishable goods.
Smart shipments and delivery
AI transforms shipping through real-time analytics, predictive capabilities, improved scheduling, automated processes, and more.
For example, Walmart’s Express Delivery, an AI-based system, features resource optimization and vehicle routing. First, it determines whether the customers are eligible for the two-hour delivery option in the first place. Then, it optimizes routes and assigns delivery trips.
UPS uses an AI-powered GPS tool called ORION (On-road Integrated Optimization and Navigation) to create routes for its fleet. The system collects customer, driver, and vehicle data and uses algorithms to create optimal routes.
AI-powered robots will be delivering more and more items.
Cybersecurity
With the growing scale and complexity of cyber threats, AI has become indispensable. Intelligence software can recognize hacking activities, ransomware attacks, other cyber threats, and holes in computer network defenses by monitoring patterns and identifying anomalies in data input. Once an AI system detects a threat, it can backtrack through the data to find the source and alert the personnel in charge. AI-infused solutions also offer self-healing capabilities for infrastructure and the prevention of future threats.
Financial services use AI and ML for fraud detection and digital and data security, analyzing historical and real-time data to make near-instantaneous decisions regarding individual transactions. Banks deploy ML models that can detect suspicious transactions nearly in real time, stop them immediately, and alert the authorities. Companies like DataVisor and Teradata provide banks with specialized AI-based financial fraud detection solutions.
Read also: 10 Best Practices to Promote FinTech Security
Business processes automation
AI can also transform a company’s operations from the inside, automating certain business processes and repetitive tasks. For instance, AI-powered bots and voice assistants can act as personal assistants to help employees manage emails and maintain calendars.
30% of global IT professionals say employees at their organization are already saving time with new AI and automation software and tools.
Businesses in most markets reportedly use or consider using automation to drive greater efficiencies in business processes, tasks, and IT operations (AIOps). IT professionals in Australia, Canada, France, UK, and the US are more likely to cite cost savings as the reason.
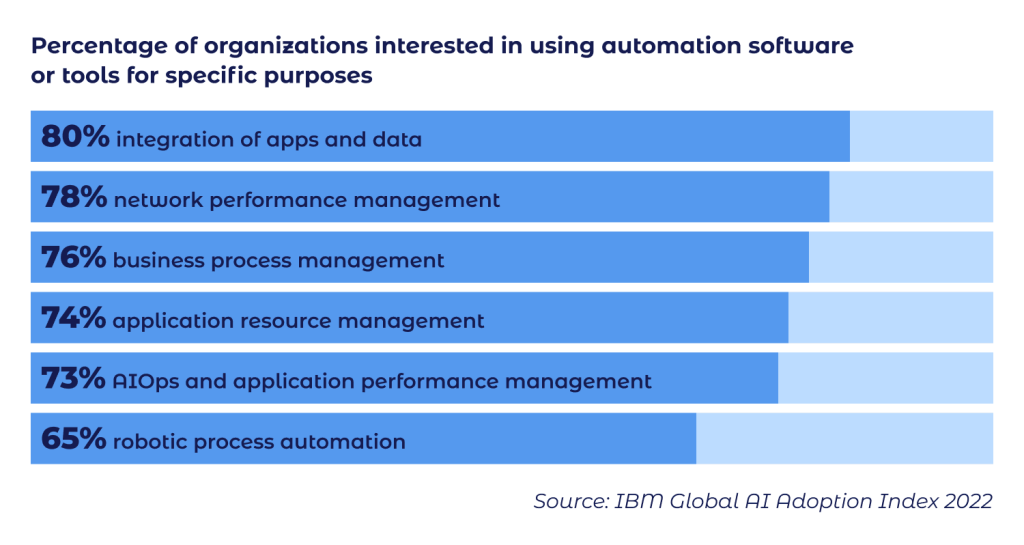
Increasing efficiency, safety, and sustainability
In 2022, one-third of companies reportedly used AI to drive more efficient business processes and operations. Companies are looking to AI to reduce costs and consumption, manage reporting, streamline operations, and solve sustainability challenges.
Optimization is another use case that stretches across industries, businesses, and processes. AI-based business applications can turn various data into actionable insights on optimizing an organization’s functions ranging from worker schedules to product pricing.
According to the MIT Sloan Management Review and Boston Consulting Group’s 2021 global study, over 75% of managers who reported improved decision-making and efficiency due to AI implementations also saw improvements in collective learning, team morale, and collaboration.
Businesses are increasingly looking to leverage AI to improve their recruiting and retention policies, employ more diverse workforces, and enhance their skills, training, and safety.
For example, manufacturing, construction, mining, utility companies, farms, and other enterprises garner data from cameras, motion detectors, thermometers, weather sensors, and other endpoint devices. A combination of AI, data from on-site IoT (Internet-of-things) systems, and computer vision enables monitoring workers’ behaviors and conditions to ensure compliance with safety protocols and quickly detect hazards. Intelligent systems can process the data to identify dangerous conditions, problematic behaviors, or business opportunities, make recommendations, or even take preventative or corrective actions.
AI also helps companies repair or replace parts or machinery before something breaks. For example, at a plant, manufacturing machinery and devices connected to a network feed a constant stream of data about functionality, production, etc., to a central location. ML systems can analyze the data in nearly real time, identifying patterns and anomalies. If a machine works at a reduced capacity, an ML algorithm can notify decision-makers that it’s time to dispatch a preventive maintenance team.
Predictive maintenance software also uses AI and massive amounts of data from sensors, historical maintenance records, and weather data to determine when equipment will need service. Operators can make better-informed decisions about machine repairs and maintenance work schedules.
Smart energy management systems collect data from sensors affixed to various assets. ML algorithms then contextualize the datasets, helping decision-makers understand energy usage and maintenance demands.
Companies also apply AI to more far-reaching challenges, such as skills or labor shortages, environmental, social, and corporate governance (ESG) initiatives, or reducing their environmental impact. 66% of companies apply or plan to apply AI to address their sustainability goals, and 64% are currently using it to accelerate their ESG initiatives.
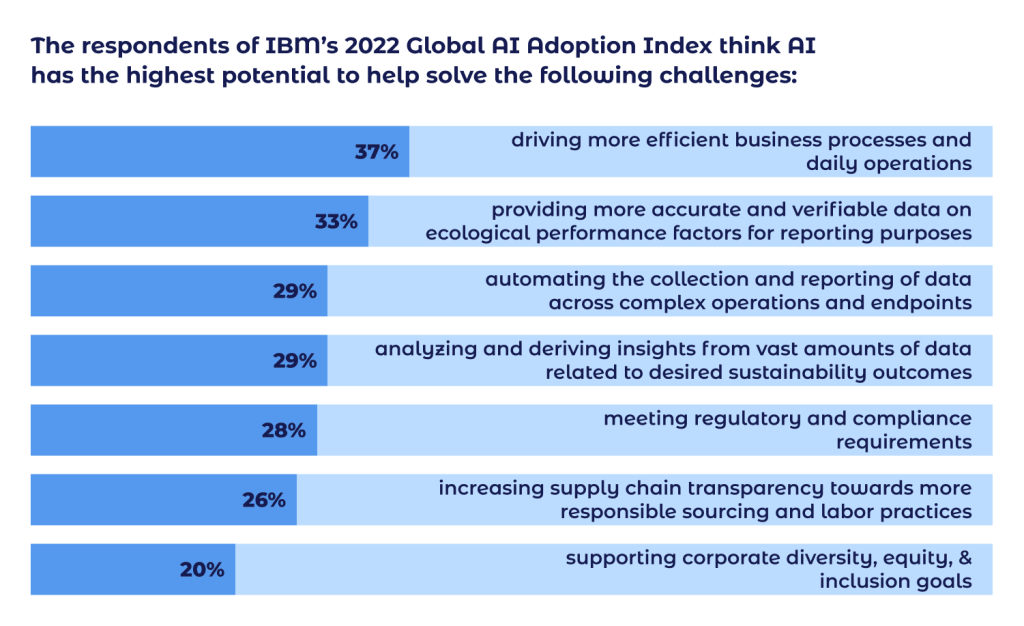
The reasons for the perceived limited use of artificial intelligence in business sectors are also varied.
The Challenges to Deploying AI for Business Needs
IBM’s 2022 Global AI Adoption Index lists five main factors hindering the successful adoption of AI for businesses’ use:
- limited AI skills, expertise, or knowledge (reported by 34% of respondents)
- the price is too high (29%)
- lack of tools or platforms to develop models (25%)
- difficulty tackling, integrating, or scaling complex projects (24%)
- excessive data complexity (24%)
Let’s take a look at these and related difficulties and how companies can alleviate them.
Limited AI skills, expertise, or knowledge
The lack of skills remains the most significant barrier to AI adoption within organizations. 19% reportedly don’t have employees with the right skills to integrate AI and ML technology into business processes and use new AI software and tools.
Data scientists and professionals with particular technical abilities may be hard to find, and there’s always someone willing to pay them more. It’s even harder to hire people with an understanding of business strategy and digital technology who can generate insights from corporate data.
Companies may respond to this challenge by training their own AI workforce. One in three organizations, primarily larger companies, are reportedly preparing and reskilling personnel to work with AI and automation software and tools. This capability and financial resources may explain the significant gap in AI adoption between larger and smaller companies.
Smaller companies, especially for projects with limited budgets, can outsource the work. Besides quick access to seasoned professionals that know all ins and outs of data science, integrating AI and ML with business applications, and other technicalities, this approach can help save up to 50% of a project budget.
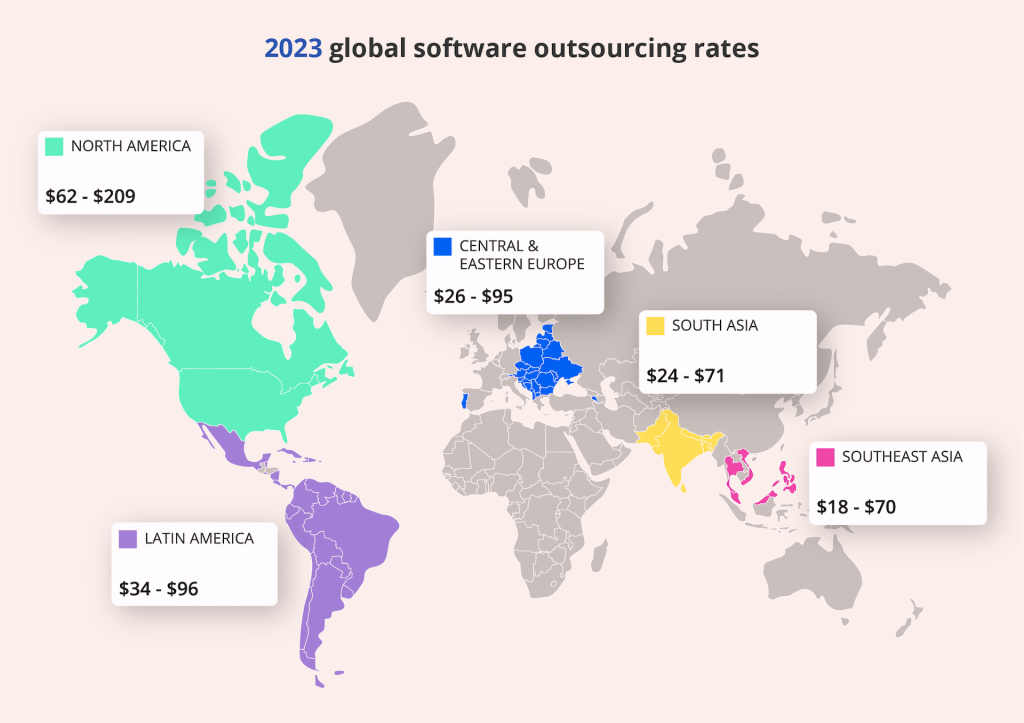
Budget issues
Deployment of artificial intelligence for business use can be long and expensive, especially when driven by big data and sophisticated technologies. For example, IT professionals perceived NLP capabilities as expensive: 54% reported cost as a barrier.
If a company ventures to build and integrate its own AI solutions, it should objectively assess the level of research and development it can sustain. AI innovation is only feasible if a company is big enough and has unique business needs, appropriate capabilities, and a strapping budget.
Still, usually, it’s enough to be fast or late followers. The AI technology market has a lot to offer already. Small and middle-sized companies can find state-of-the-art AI solutions that are open-source and available for free or pay for solutions developed by Amazon and other AI champions based on usage. As AI matures, the technology and necessary expertise will become increasingly accessible. More vendors will be replicating successful AI applications and offering products at increasingly affordable prices.
The development and maintenance of custom AI systems require massive computing power, a modern networking infrastructure, data engineers, and skillful project management. AI-compatible infrastructure must be flexible and scalable and have the sufficient data processing power. Enterprise-level computing resources are expensive.
The good news is that cloud-based services eliminate the costs of bringing in new servers and powerful processors. AI can also be made more affordable through partnerships with universities and tech firms possessing the knowledge and infrastructure for building robust AI models.
If a whole in-house team looks like too big an investment, an experienced dedicated team can be a perfect solution.
Data management difficulties
ML powering most AI applications requires vast amounts of data to train the models. Most companies worldwide reportedly draw from over 20 different data sources to inform their AI, business intelligence, and analytics systems.
This limits the use of artificial intelligence in business areas that are new or where there isn’t much data available. Smaller companies are three times as likely as large organizations to have fewer than 20 data sources.
Without sufficient clean data sets, ML algorithms’ ability to learn and analyze will be limited. However, data still largely comes unstructured and unlabeled.
Training an ML algorithm on biased data will result in inaccurate results. For example, experts once found Amazon’s Rekognition system to perform more accurately when assessing lighter-skinned faces. Such biases will be properly addressed in the future, but for now, they pose a barrier to the adoption of AI in areas like security and law enforcement.
AI requires more sophisticated cloud architecture and more complex data architecture. For example, businesses that use AI are more likely to utilize a data fabric, i.e. architecture that facilitates the end-to-end integration of various data pipelines and cloud environments through the use of intelligent and automated systems. Companies that have deployed AI are 65% more likely to use a mix of architectures, including databases, data lakes, data warehouses, and data lakehouses than those that have not.
AI initiatives involving the collection of sensitive information raise additional concerns about data security and privacy. 1 in 5 IT professionals across most countries cites the following challenges to their organization’s data management strategy:
- ensuring data security
- ensuring data governance
- managing disparate data sources and formats
- integrating data across a cloud
Where to apply AI
The primary way to reduce the cost and risks of AI-powered digital transformations is to select the right task for AI in your organization.
AI is not a one-size-fits-all solution. Business leaders must think independently about where it may benefit their company most and how it can fit into their business model. Their decisions should not be formed by vendor companies, consulting firms that want enterprise deals, or hype-mongering tech media. They should also recognize the complexity of AI deployment and manage expectations.
Larger companies are 60% more likely than smaller companies to have a holistic strategy for using AI across their organization. They have dedicated groups in place for formulating business problems for AI and proving out hypotheses. These groups may create techniques to manage the data pipeline, provide training across the enterprise, and more. Other businesses often look for data scientists elsewhere.
Before investing in any AI applications, it’s essential to identify the most pressing issues and determine whether AI is the right solution. Learn what is viable and what is possible. Try to predict the AI solution’s possible impact on customers and employees.
To identify problems that AI can solve, it’s helpful to familiarize your entire team with its types and capabilities. Help them understand what machine learning and deep learning mean, what data they require, what AI solutions can do for them, etc. Otherwise, your employees might never approach the AI team with a problem or idea.
Find out who uses AI solutions for your kind of problem. Browse your competitors’ websites, social media, press releases, news coverage, and blogs to figure out how they’re putting AI technology to work. Try to learn what it takes to implement such AI applications and what to expect. When communicating with vendors, ask them what companies like yours are clearly delivering ROI from their AI solutions.
Cultural challenges and lack of trust in AI technology
The promise of AI can be delivered only when its use is trusted, supported, and safe. A company’s leaders must believe in the potential of AI for business use and be willing to facilitate changes, but AI-related decisions require conversations and cooperation on all levels. A corporate culture that promotes experimentation and more dynamic and scalable environments is the best for AI implementation.
It is also a mistake to restrict the task of AI implementation solely to the IT department. Successful deployment of an AI solution requires people running daily business processes to contribute and actively participate throughout the process. In data-driven companies, data scientists should act as links between all departments.
There are also decades-old concerns about privacy and security arising from AI development and expectations that AI will replace jobs.
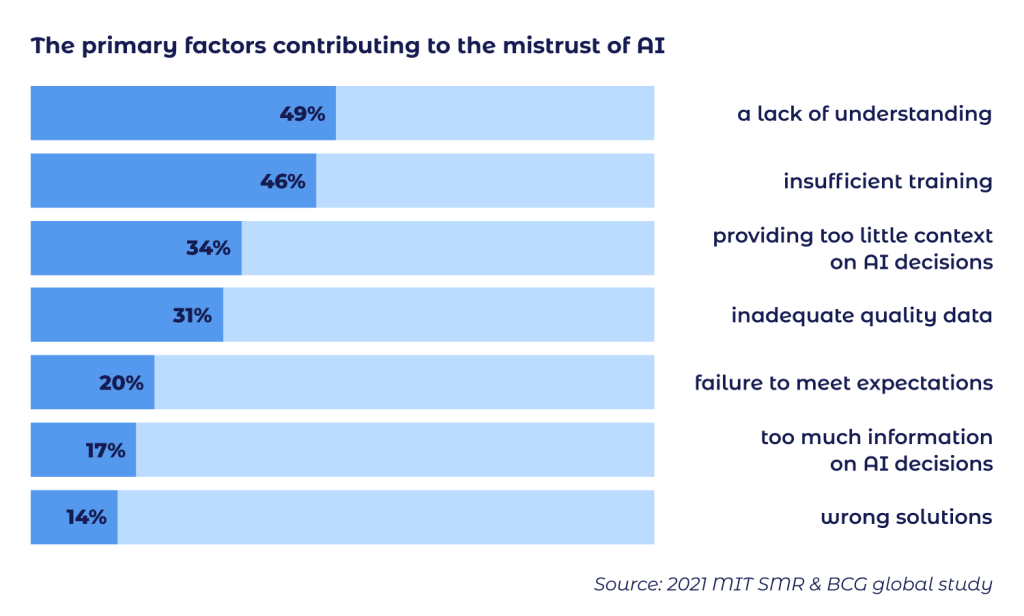
Instead of fearing losing their job, every employee should be aware of the benefits of AI and how it will make their work easier. Simultaneously, the company should implement and publicize the necessary cybersecurity measures designed to protect consumer and corporate data.
Extended timeline
After the initial planning, research, and development, time will be required to tailor and configure an AI solution to your business and knowledge domain. The integration of a custom-built AI system into your business processes and IT architecture will require additional time for adaptation. You will also have to redesign the business processes around the AI solution.
A lot of initial training and work with data is required before an AI solution can work with the existing system and deliver tangible results. Afterward, subject matter experts will have to monitor it to ensure the machine interprets the change in the business context correctly.
Fully autonomous AI systems are rare. That will typically mean new roles for the employees who work alongside them. Retraining workers on the new process and system may require considerable time again.
Even a fully autonomous AI system is likely to require some augmentation. During this period, the interaction between the system and the users and observers should occur. The collection of new data sets and baking them into ML algorithms may take months on end.
Uncertain value of AI applications in business
It’s difficult to measure and predict the ROI because few companies implement AI at a scale sufficient to generate substantial financial benefits. Moreover, improvements in quality and efficiency brought about by AI and machine learning may be visible only in the long run.
Even AI that is effective at the team level doesn’t always yield financial success at the organizational level. The MIT SMR-BCG 2021 global study revealed that 58% of the surveyed executives saw their teams’ efficiency and decision quality improve since implementing AI solutions. However, only 11% saw significant financial benefits from their AI initiatives.
Ethical concerns
Building ethical AI is a complex task. For example, lawmakers worldwide struggle with regulating government and commercial use of facial recognition technology and continue investigating its possible risks. For instance, mistaken identification of a person as someone else may be fatal sometimes, while facial recognition systems can be less accurate when trying to identify people with darker skin.
Facial and voice recognition technologies also raise the sensitive issue of privacy. AI, in general, can bring the analysis of personal information to new levels that can intrude on privacy interests.
The risk of reinforced societal biases has prompted calls for greater transparency about ML decision-making. As its adoption grows, especially in the government or public sectors, the ability to explain, audit, and understand how an AI agent arrived at its decisions or classifications will become increasingly important. A staff member responsible for ethical AI implementation and accountability may become mandatory for businesses that use AI.
Other ethical concerns include the possible rise of fake media and disinformation, security holes and other vulnerabilities in AI systems, and the ability to manipulate or fool them.
The challenges faced by artificial intelligence-related businesses or companies that wish to use AI are many and various, yet more and more companies seem to overcome them. Here are several AI implementation success stories.
Businesses that Have Transformed Their Operations with AI
Alibaba
Alibaba earned over 207.18 billion yuan (29.7 billion USD) in Q3 2022.
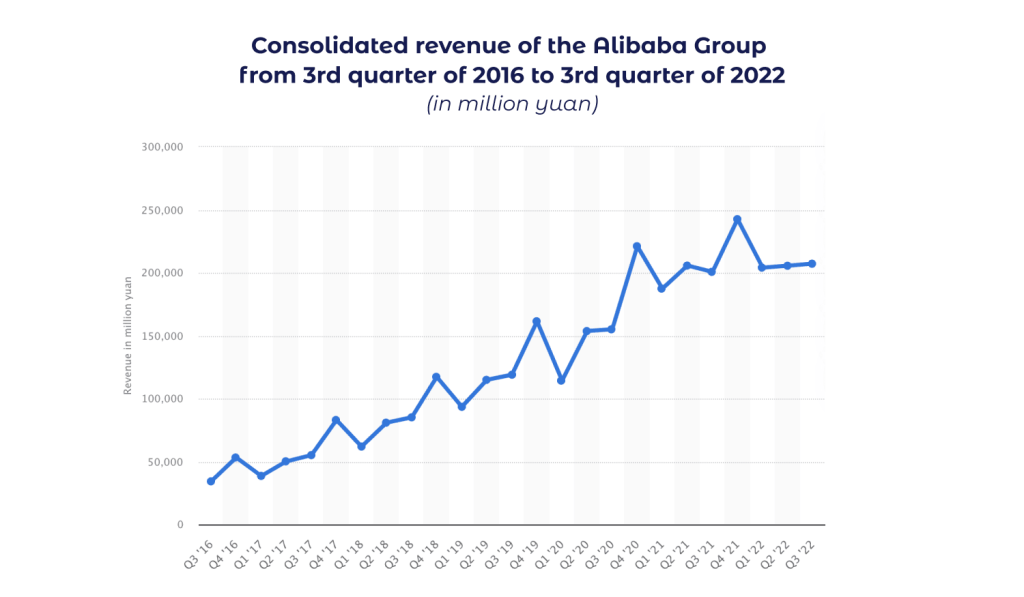
Its active research and implementation of AI and ML solutions are evident in
- a $15 billion investment in its global R&D DAMO Academy in 2017, including in research areas that focus on data intelligence, IoT, human-machine interaction, and quantum computing;
- a $600 million investment in SenseTime, a deep learning and computer vision company, in 2018;
- a $1.41 billion investment in its AI and IoT system centered around smart speakers in 2020;
- a 2021 investment in the autonomous driving startup DeepRoute.ai.
Even the world’s largest ecommerce platform is preoccupied with offering the most relevant products and enhancing the online shopping experience on its Tmall website and mobile app.
Managing traffic from nearly 1.3 billion users while optimizing the shopping experience requires intensive use of ML and cloud AI functions. Alibaba’s Recommendation System Framework (RSF) was designed to optimize traffic flow and promptly offer consumers the products they want, increasing customer satisfaction, click-through rate, and revenue.
Before 2019, Alibaba used a “relevance of recommendation” function that calculated the degree of similarity between the products previously clicked on or purchased with Tmall’s inventory. The company changed it to include the diversity of recommendations and discovery optimization using the Artificial Intelligence Recommendation (AIRec) engine.
AIRec algorithms outperform “self-managed” algorithms by 20-100%. The engine can analyze and capture user behavior in seconds and within milliseconds, provide personalized recommendations for more diverse products with a high click-through rate.
Anheuser-Busch InBev
AB InBev is the largest brewer of beer, its brands including Budweiser, Corona, Hoegaarden, Stella Artois, etc. Its revenue for the 12 months ending September 30, 2022, was 57.317 billion USD, an 8.4% increase year-over-year. The company operates in 50+ countries. Started in 1366, it might seem an unlikely digital transformations pioneer, but it is.
Tassilo Festetics, then AB InBev’s vice president for global solutions, advised: “Really start looking at your data early, because data is the fundamental part. […] Then start looking at the areas where you have the best business cases, where can you drive the most value for your company.”
They began in 2013 by collaborating with farmers through the Smart Barley platform. Its AI algorithm analyzes data from past yields to help improve future yields, reduce water and fertilizer usage, and create a more sustainable environment.
Then, AB InBev brought analytics, AI, and predictive modeling to their back-office operations and the supply chain with the help of Microsoft Azure and Google Cloud Platform’s services. By optimizing its data pipeline and deploying AI solutions from Azure, AB InBev cut down on shipping times and fuel costs.
Google Cloud Platform helped AB InBev enhance its beer filtration process. They used AI and ML on data collected from sensors in the filtration equipment to find the sweet spot for filtering the finished product. This resulted in lower filtering costs and a competitive advantage. AB InBev then deployed an AI solution for maintaining brewing machinery.
AB InBev established a Growth Analytics Center in Bangalore, India. Its data scientists use AI and Azure ML capabilities to look at current and historical data from multiple sources. They develop models used for forecasting and identify patterns that can help optimize prices and promotions and achieve the highest possible customer retention rates.
Founded in 2006, Twitter quickly developed into an alternative to Facebook.
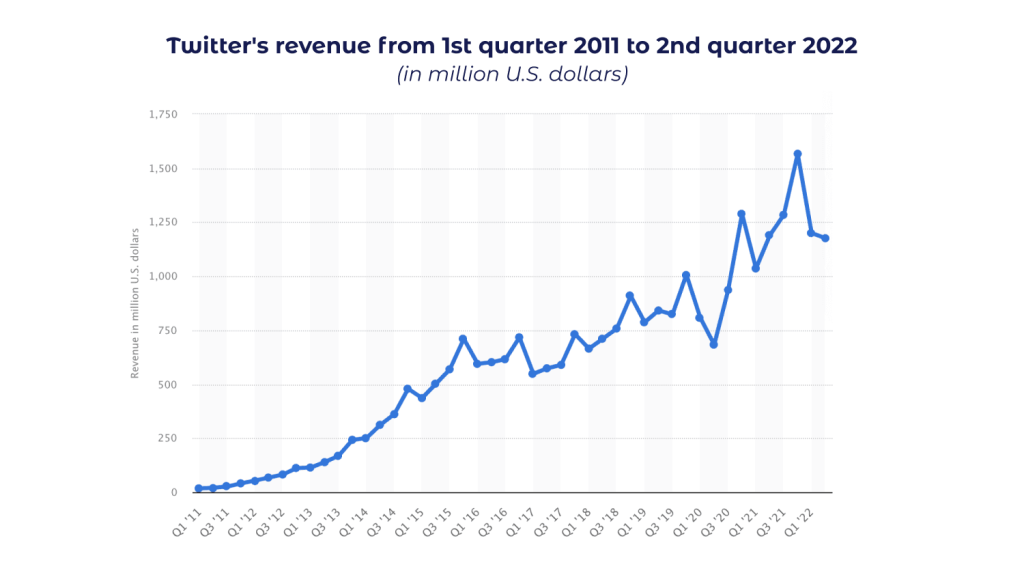
However, as the platform grew, it saw an increase in hate speech, fake news, and terrorism-related and illegal content.
To mitigate possible legal and social implications, Twitter started leveraging AI and ML tools to transform its content management. For example, the company acquired
- the image search startup Madbits in 2014
- machine learning startup Whetlab in 2015
- Magic Pony Technology that used neural networks to improve images (2016)
- Fabula AI using ML to detect fake news online (2019)
As a result of these and other efforts, Twitter’s algorithms now detect and remove incidents of hate speech, illegal, racist, and other offensive content. In the first six months of AI usage, Twitter was able to eliminate 300K accounts related to terrorists or promoting such activities.
AI is also used to crop images in a specific way, offering users a nearly accurate crop that helps drive engagement. If the system detects adult content in an image, it displays a message but hides the image. Video recognition software facilitated an accurate categorization of videos, improving their searchability.
Showing relevant tweet suggestions to every user was another challenge. Twitter’s timeline is currently driven by a complex deep learning algorithm powered by deep neural networks. It runs millions of tweets and reactions to them through these systems to determine which users want to see what type of content.
AI algorithms scan and score every tweet to determine whether a user would like to see it. The ranking model considers the tweet’s content, the number of likes and retweets, and any connection or previous interactions between its author and the user. A tweet with a high relevancy score will likely appear in the user’s feed.
Alternative-spaces also has experience in Twitter sentiment analysis and news categorization and built an NLP-powered auto-reply assistant that can keep a conversation on a social network when the user is offline.
Alternative-spaces has also helped its clients implement AI solutions for their business needs. For example, our mobile developers have recently launched an AI-powered solution for the cosmetics industry.
With thousands of beauty and skincare products on the market, it’s easy to be confused and make costly mistakes. Our client came up with a big idea: a one-of-a-kind skincare-focused AI-powered platform and mobile app.
The iOS app is designed to help users:
- create skincare routines for their unique skin type and needs based on scientific data and up-to-date regulatory resources
- easily find safe beauty products suitable for their age, skin type, needs, and lifestyle
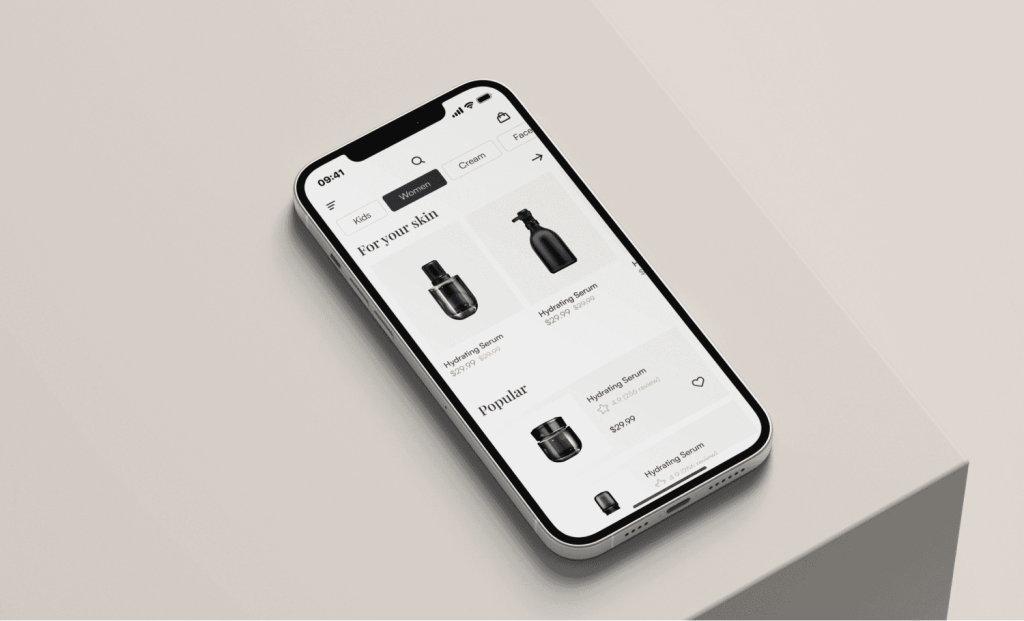
The work began in 2019. The project included the creation of a massive ingredient database and an AI-powered skincare and cosmetics analysis tool. With the help of ML technologies, Alternative-spaces’s experts enhanced one of the largest global ingredient databases that helps understand products’ benefits, toxicity, and other characteristics. They also developed a classification system for all cosmetic products on the consumer goods market. Numerous data scrapers assisted in obtaining information from the Internet, scientific literature, and PDF articles.
After a user fills in a questionnaire, the science-backed AI-powered recommendation engine analyzes 800,000+ ingredients to find the most suitable product. Users can quickly search for desired cosmetics across thousands of brands and compare prices. Personalized skincare recommendations and safety ratings help them buy safe and effective beauty products from verified vendors.
The results to this date include:
- 100,000+ Ingredients cataloged
- 200,000+ product formulas benchmarked
- 45,000+ products analyzed
- 100,000+ happy customers
The collaboration helped our client achieve a leading position as an ingredient transparency solution for the cosmetics industry.
Conclusion: What Business Can Expect from AI in the Future
Amazon, Alibaba, Facebook, Google, Netflix, Spotify, and Twitter likely would not have become global leaders without machine learning. Banking, food, pharmaceutical, and automotive industry giants, and virtually all tech companies have also been using AI to great effect.
The primary AI applications in business include data analytics, personalized business offerings, and automation. Existing AI solutions already have the potential to transform marketing, sales, customer services, IT, human resources, decision-making, administration, finance, manufacturing, quality control, workplace safety, and cybersecurity.
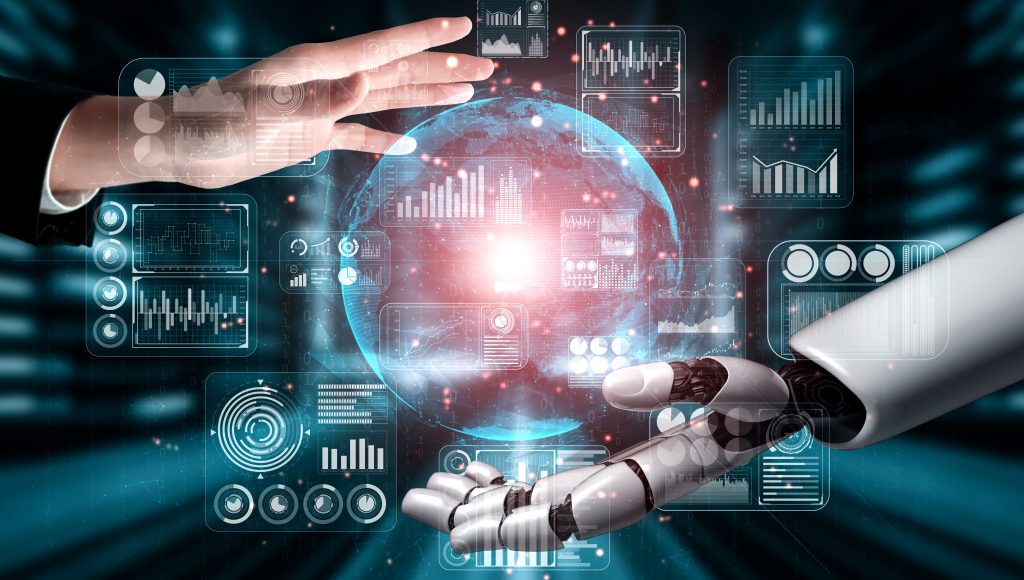
Slowly for innovators and too fast for laggards, the artificial intelligence impact on business will grow. Successful organizations have moved beyond using AI to improve their current processes to uncover new business opportunities to create a competitive advantage.
Although powerful and impressive, today’s AI is still only a statistical analysis method. Real-world AI systems are typically only capable of singular tasks requiring human intervention to support and apply the technology in the workplace. There’s much work to be done, such as reducing unintended bias, etc. However, “commonsense” tasks are becoming easier for computers to process. Graphic processing units (GPUs) will become faster, improving the applications of AI software across businesses.
AI is expected to move increasingly from two-dimensional screens to the three-dimensional world. The combination of AI with IoT, virtual reality, augmented reality, and other technologies will replace traditional displays as the primary interface; the environment surrounding workers will become one. New user interfaces, digital assistants, and robots will proliferate across industries and businesses.
Researchers aren’t sure what AI means for white- and blue-collar jobs in the future. Some experts worry that AI will automate so many jobs as to steal them from millions of people, such as drivers and assembly line positions, or replace business analysts, hedge fund managers, and similar white-collar jobs. Others deny that possibility.
Some even theorize that widespread AI adoption will create more jobs across different sectors in the coming years, if not decades. For example, in its 2020 Future of Jobs Report, the World Economic Forum estimated that AI would replace 85 million jobs but would cause some 97 million new jobs to emerge by 2025.
One can compare this with the previous revolutionary shifts driven by computers, electricity, or cars. The shift toward AI-based systems will fragment long-standing workflows; new jobs will be required to facilitate that transition, integrate those workflows, and support innovation.
AI-driven massive innovation will fuel many existing industries and may even create new sectors for growth. We will see new startups, business applications, and consumer uses. AI may help in the creation of a knowledge-based economy and, through automation, a better way of life. We’re just scratching the surface of what AI can do and can become.
The future is coming quickly, and companies that don’t want to miss out on that revolutionary future must embrace AI and big data as early as possible. The playing field will only become more competitive. By the time a late adopter is poised to leverage AI, early adopters will be operating at lower costs with better performance. Laggards may never be able to catch up.
If you anticipate a threat from AI-driven competitors or new entrants, it’s best to start exploring AI’s abilities now. If you don’t know how to implement AI in your business, ask for expert advice. Please don’t hesitate to contact Alternative-spaces – we can provide a consultation, offer software engineers to hire, or build an AI solution for your business from A to Z.
Frequently Asked Questions
What applications of artificial intelligence in businesses are developing now?
- business intelligence (forecasts and predictions)
- personalized customer experiences, service, and support
- smart searches, product recommendations, and purchase predictions
- ad targeting and customer segmentation
- content recommendations, curation, and feed personalization
- supply chain optimization
- pattern, voice, and image recognition
- chatbots and digital assistants
- cybersecurity and fraud detection
- business processes automation
- workplace safety
- predictive maintenance
- route optimization and delivery by robots
What are the primary barriers to the use of artificial intelligence in business?
- a costly but limited AI talent pool
- ROI uncertainty
- mistrust of machine intelligence
- difficulty tackling, integrating, or scaling complex projects
- insufficient training data and data management issues
- limitations in current computing capability
- threats to data security and concerns about privacy
- ethical concerns, including biases and possible loss of jobs
Are there examples of successful application of artificial intelligence in business to date?
Some prominent businesses that use AI to the greatest effect are Alibaba, Amazon, Google, IBM, Meta Platforms, Microsoft, Netflix, Salesforce, and Starbucks.
Content created by our partner, Onix-systems.
Source: https://onix-systems.com/blog/artificial-intelligence-in-business-meeting-the-challenges-to-ai-adoption