Artificial Intelligence in Business: Meeting the Challenges to AI Adoption
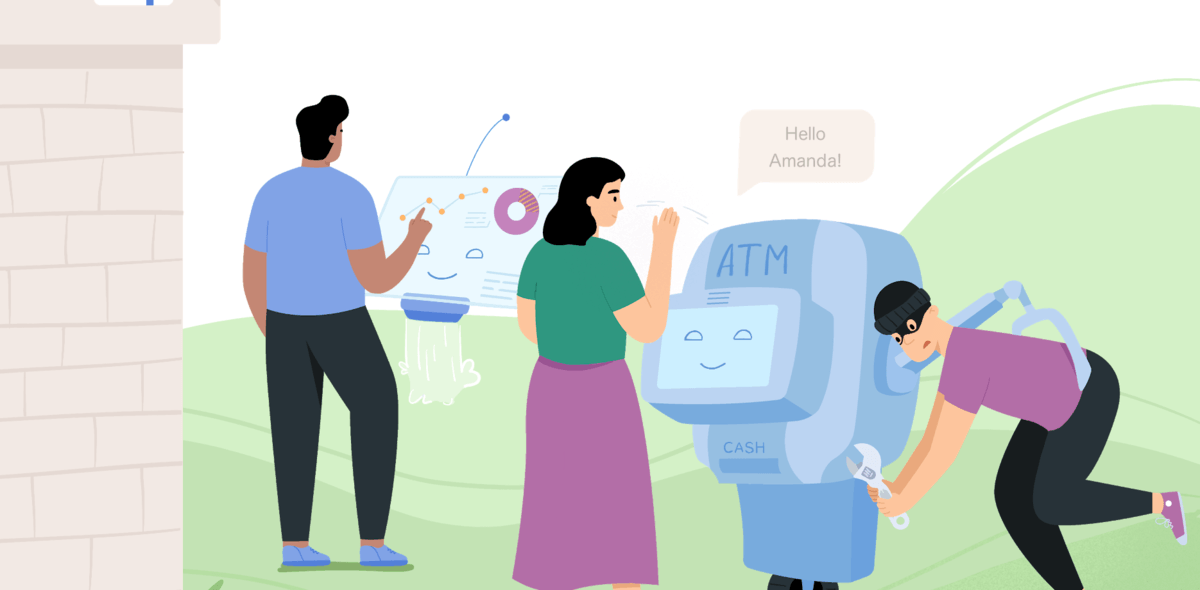
Artificial intelligence (AI) is often and justifiably labeled as something revolutionary and game-changing. Amazon, Facebook, Google, or Netflix would not have become what they are today without machine learning. Large banks, pharmaceutical and automotive industry leaders, and virtually all tech companies have been using AI to great effect too. According to the 2019 RELX Emerging Tech Executive Report, the understanding of the benefits of AI and hence its use continue growing.
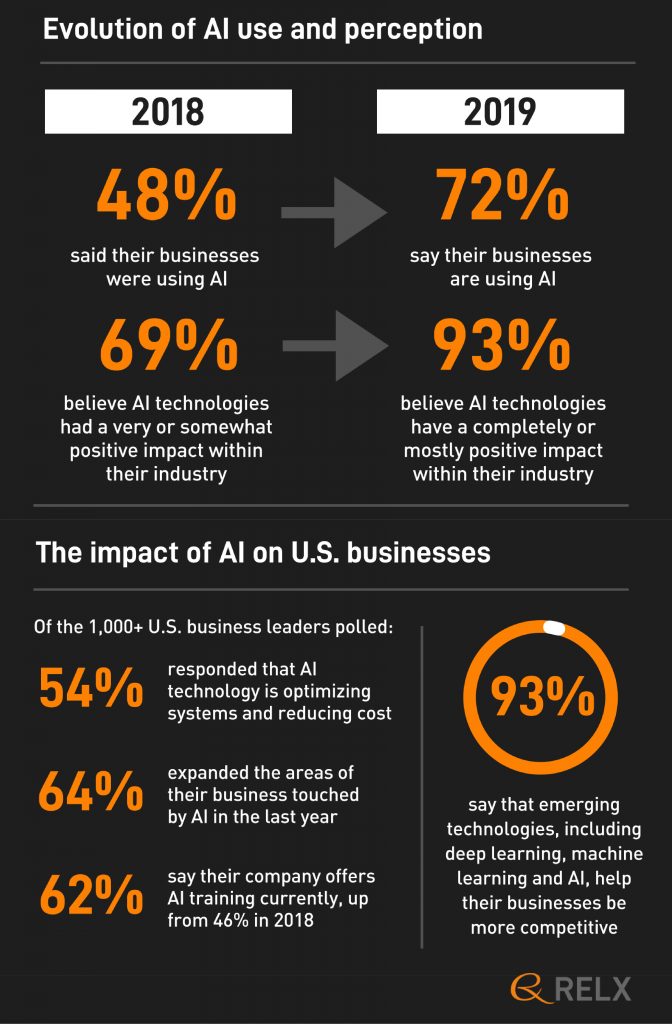
However, according to Gartner’s 2019 CIO Agenda Survey, only 37% of the respondents said that they deployed AI technology or that the deployment was in short-term planning. It looks like only big companies have been able to capitalize on AI applications. Smaller entities still either have them in the “pilot” phase or regard AI as a far-off goal.
The reasons for the perceived inadequate progress in adopting AI for business processes are varied. For example, according to the same RELX Report:
- 50% of companies that have not yet adopted AI cite budget constraints as the primary reason;
- 36% complain about the lack of technical expertise;
- 30% cite unproven return on investment (ROI);
- 16% lack the appropriate C-suite or Board buy-in.
We might also add misconceptions to this list. Let’s take a look at these and related difficulties and how companies can alleviate them.
The Challenges to Deploying AI for Business Needs
The Budget Issues
Let’s say upfront: unless a company is big enough and has a unique set of business needs coupled with appropriate capabilities and a strapping budget, there’s no need to plunge into expensive AI innovation at all. Usually, it’s enough to be fast or late followers. The market of AI technology has a lot to offer already. Small and middle-sized companies can find state-of-the-art AI solutions that are open-source and available for free, or pay for solutions developed by Amazon and other big AI innovators based on usage. As AI matures, the technology and necessary expertise will become increasingly accessible. More vendors will be replicating successful AI applications and offering AI solutions at competitive prices, making them increasingly affordable.
If a company ventures building and integrating own AI solutions, it should objectively assess the level of research and development it can bear both psychologically and financially. The development and maintenance of AI systems involve a project manager, data engineers, and modern networking infrastructure.
AI systems become smarter only by processing vast amounts of data. AI-compatible infrastructure has to be flexible and scalable and have sufficient data processing power. The good news is that cloud-based services eliminate the costs of bringing in new servers and powerful processors. AI can also be made more affordable through partnerships with universities and tech firms possessing the knowledge and infrastructure for building robust AI models.
If a full in-house data science team looks like too big an investment, the problem may be solved by outsourcing the AI project.
AI projects can eat up a portion of a company’s resources, but digital transformations always come at a cost. At least you can reduce the risks by selecting the right task for your AI pilot project.
Where to Apply AI
The coverage of artificial intelligence in business media sometimes gives the impression that AI is a one-size-fits-all solution and “everybody does it.” Business leaders need to think independently about where AI may benefit the company and to understand how it can fit into their business model. Their decisions should not be formed by vendor companies, consulting firms that want enterprise deals, or tech media interested primarily in clicks and shares. They should also recognize the complexity of AI deployment and manage expectations.
The leadership should identify the most pressing areas and determine whether AI is the right solution in the first place. Learn what is viable and what is possible. For example, a small firm may have a big idea but fail at implementing it due to a lack of company data. It’s also important to predict the AI solution’s possible impact on customers and employees.
According to the same RELX Report, 57% of the companies surveyed reported that AI is helping to improve and develop products. 54% said that AI helps in optimizing control and collaboration. Gartner’s 2018 survey revealed that AI was predominantly used either to boost the customer experience or to fight fraud.
However, it’s wiser to start with tasks that are not customer-facing and business-critical. For example, you might first accelerate and reduce human error in back-office tasks like data entry, processing, and sorting. Start small and scale up as you go.
Big companies have dedicated groups in place for formulating business problems for AI and proving out hypotheses. These groups may also create techniques to manage the data pipeline, provide training across the enterprise, and so on. Other businesses often look elsewhere for data scientists with a deep knowledge of their specifics. However, it may be better to teach in-house experts to identify problems that AI can solve.
Familiarize your staff with the types of AI technology. Let them learn, e.g., what deep learning means or what data natural language processing requires, and which task each one is good for. For example, AI-based cognitive engagement can collect information, provide a more personalized customer experience, and help solve issues in real time. AI-powered chatbots may enhance your customer service. Predictive recommendations like Amazon’s or Spotify’s can help dramatically improve customer engagement and ROI. Data analytics gives the management the predictive power to make informed decisions and jump on trends before they happen. Artificial neural networks power multiple business cases too.
Find out who is using AI solutions for your kind of problem. Browse your competitors’ websites, social media, press releases, news coverage, and blogs to figure out how they’re putting AI technology to work. Reach out to companies of your size with successful AI applications to learn what it takes to implement such things and what to expect. When communicating with vendors, ask them what companies similar to yours are clearly delivering ROI from their AI solutions.
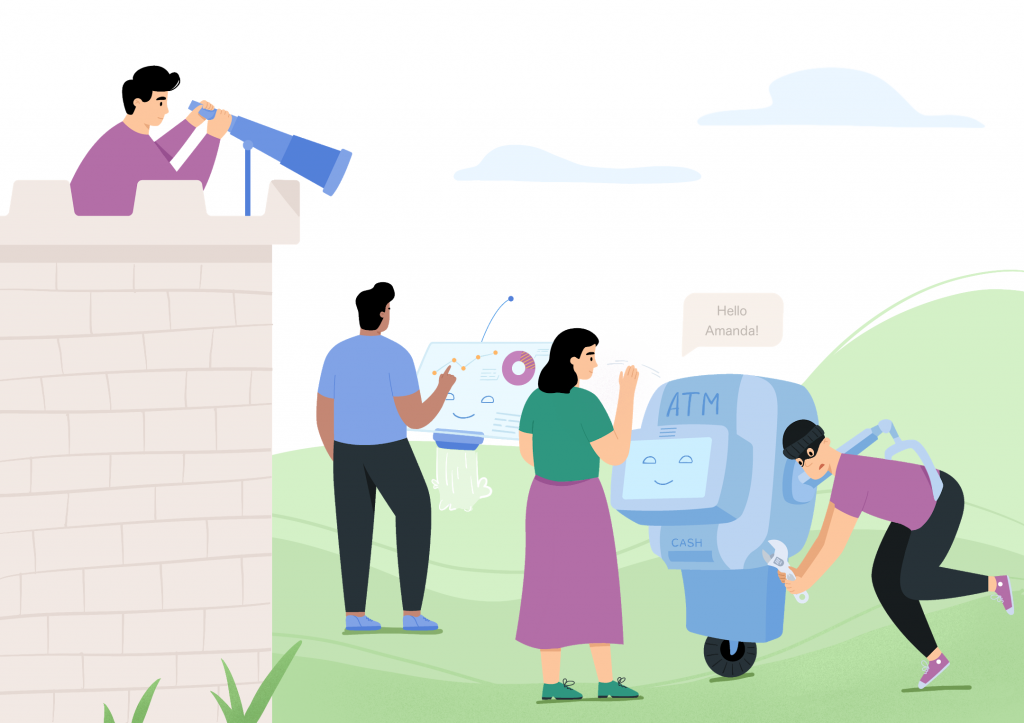
Cultural Challenges
It’s crucial that a company’s leaders believe in the potential of AI for business and are willing to learn new methods and facilitate changes. However, AI-related decisions require conversations and cooperation on all levels. A corporate culture that promotes experimentation and more dynamic and scalable environments are the best for AI implementation.
Many organizations charge the IT department with the task of AI implementation. That is a mistake. Every employee should be aware of and look for the benefits of AI to be realized in their job. Otherwise, companies risk underutilizing them. For instance, if the marketing team doesn’t know what machine intelligence can do for them, they may never approach the AI team. Successful deployment of an AI solution requires that people running daily business processes should contribute and actively participate throughout the process. In data-driven companies, data scientists should act as links between all departments.
The adoption of AI into an organization’s environment may take significant research and development effort. A lot of initial training and work with data is required before an AI solution can work with the existing system and deliver tangible results. Afterward, monitoring by subject matter experts will be required to ensure the machine is interpreting the change in the business context correctly.
Lack of Technical Expertise
According to Gartner’s 2018 survey, when CIOs surveyed ranked AI as the most problematic technology to implement, the most common complaint was that it demands new skills. Data scientists and professionals with particular technical abilities may be hard to find, and there’s almost always someone willing to pay them more. It’s even harder to hire people with a firm understanding of business strategy and digital technology who can generate insights from corporate data.
Companies may respond to this challenge by training their own AI workforce. According to the 2019 MIT Sloan Management Review and Boston Consulting Group AI Global Executive Study and Research Report, companies that support their existing workforces in gaining AI skills are by 40% more likely to have generated value from AI compared with companies that don’t. Unfortunately, investment in such training doesn’t seem to match the jump in AI usage. Only 62% of executives surveyed by the 2019 RELX Report said their company offers AI training. However, it’s a jump up from 46% in 2018. Of those whose companies don’t offer training, 53% say they plan to do so in the future. 93% believe that U.S. companies should invest in the future AI workforce through university partnerships and other educational initiatives.
Extended Timeline
After the initial planning, research, and development, time will be required to tailor and configure it to your business and knowledge domain. If your machine learning application manipulates language, it can be even more difficult to put in operation.
The integration of the built AI system into your business processes and IT architecture will require additional time for adaptation. The transition from prototypes to production systems can be time-consuming. You will also have to redesign the business processes around the AI solution.
Fully autonomous AI systems are rare. That will typically mean new roles for the employees who work alongside them. Retraining workers on the new process and system may require considerable time again.
Even a fully autonomous AI system is likely to require some augmentation. During this period, the interaction between the system and the users and observers should occur. The collection of new data sets and baking them into machine learning algorithms may take months on end.
Uncertain Value of AI Applications in Business
According to the same 2019 MIT SMR-BCG AI Report:
- 65% of executives worldwide report that they are not yet seeing value from the AI investments they have made;
- 40% of organizations making “significant investments” in AI do not report business gains from AI;
- only 50% of organizations across maturity groups that have invested in high-risk projects have seen value to date.
It’s difficult to measure and predict the ROI because most companies are only trying AI applications. Moreover, AI and machine learning bring about improvement in quality and efficiency which may be visible not immediately but in the long run.
The goal and the most significant outcome of AI deployment should be the improvement of people’s lives. For example, AI-powered voice user interfaces will facilitate personalized and emotional user experiences, and digital assistants may soon be recognizing customers by face and voice across channels and partners.
Why You Need to Employ AI Applications in Business, after All
Deployment of artificial intelligence for business use can be a long and expensive affair, especially when driven by big data and sophisticated technologies. A costly but limited AI talent pool, ROI uncertainty, and insufficient data are the most common obstacles on this path. However, organizations that overcome these barriers can rely on AI’s power to improve processes, increase employee satisfaction and productivity, and develop a competitive edge.
Before investing in any AI applications, it’s essential to determine whether it’s the best way to achieve your business goals and strategic objectives. The C-suite should shape the company’s AI strategy and support the culture of experimentation and relevant education and training.
The need for progress should be their motivation. Slowly for innovators and too fast for laggards, but the artificial intelligence impact on business will be growing. A range of existing AI solutions already has the potential to transform marketing, customer services, IT, human resources, decision-making, administration, finance, and cybersecurity.
If you anticipate a threat from AI-driven competitors or new entrants, it’s best to start exploring AI’s abilities now. Although the full potential of AI in business is unlikely to be adequately realized for another three to five years, don’t wait till it’s too late. By the time a late adopter is poised to leverage an AI solution, early adopters will be operating at lower costs with better performance. Companies that lag in deploying AI may never be able to catch up.
Content created by our partner, Onix-systems.